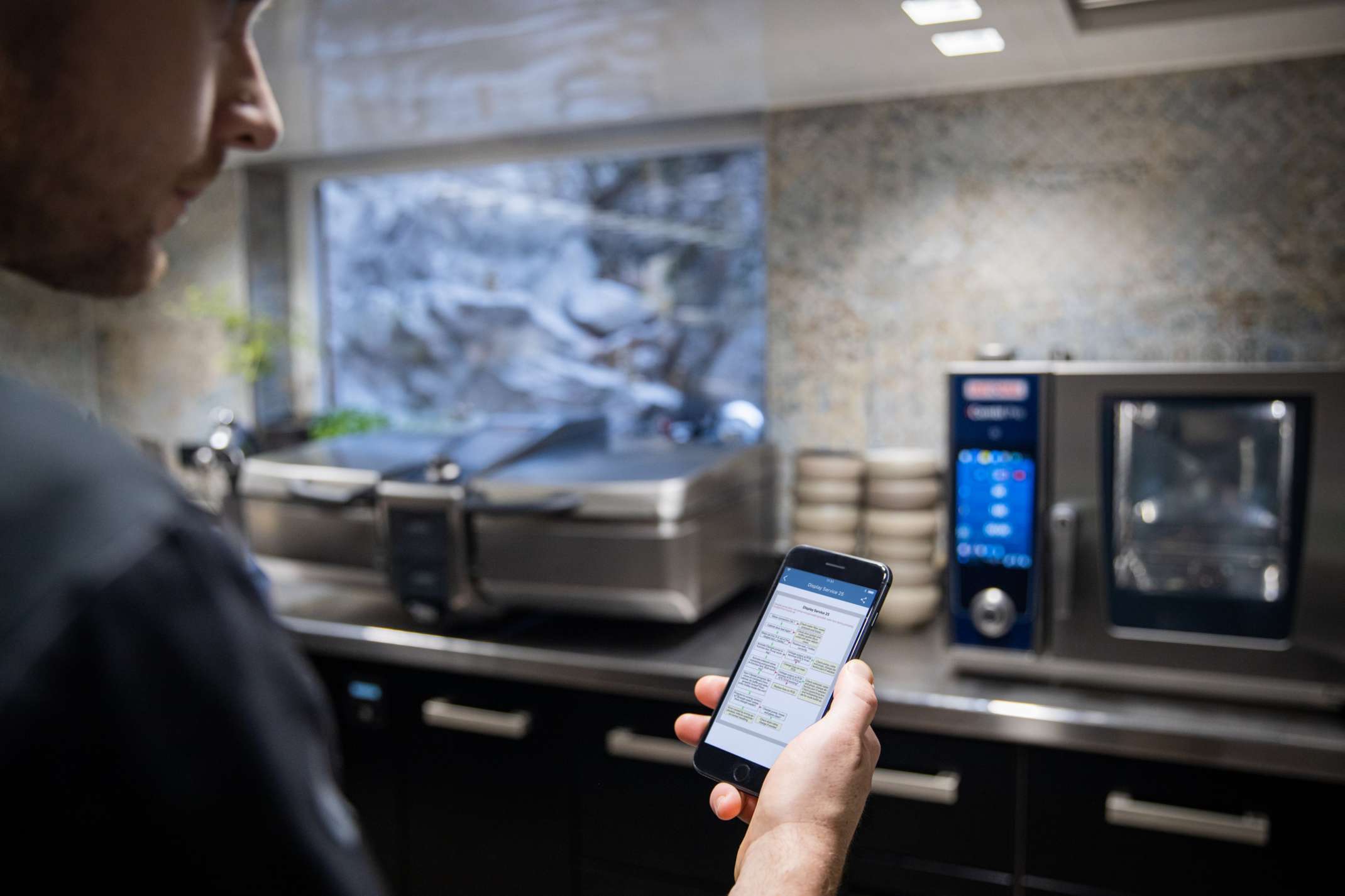
Project description
Predictive maintenance optimizes the maintenance of machines by preventing unplanned downtime and unnecessary repairs. The implementation is made possible by modern machine learning methods and a well-developed data infrastructure. In the research project PAS4PCM – in cooperation with Rational AG and on the basis of collected device data – the research group HSA_dsg of the Faculty of Computer Science at the Technical University of Augsburg is developing a concept and an implementation of such a system.
Terms
Predictive maintenance refers to the endeavor to optimize the maintenance or repair of machines using predictive models. Two aspects can be considered: Time optimization and cost optimization. Making a repair before the machine actually breaks down prevents unexpected and potentially lengthy downtime. Carrying out the repair as soon as possible before a forecast failure saves material and costs, since machines and devices can be used for as long as possible. The implementation of predictive maintenance is possible using modern machine learning models and mature Internet-of-Things infrastructures.
Cooperation
The PAS4PCM project was made possible by the cooperation partners of the Rational group and the research group Distributed Systems (HSA_dsg) at the Technical University of Augsburg. The project is part of the Bavarian joint research program (BayVFP) of the Free State of Bavaria in the „Digitization” funding line.
The research project aims to work out how a predictive maintenance solution for machines in the area of commercial kitchen appliances can be implemented. In this way, you can work together with the device data collected from Rational combi-steamers in a practical way. The goals of the research project include the development of a concept specifically for commercial kitchen appliances, the prototypical implementation of individual use cases for validation and documentation through scientific publications. The Rational devices benefit from the research in the long term, since the maintenance of the devices can be made much more efficient by implementing predictive maintenance.
Concept
A concept for implementing the solution has already been drawn up. The implementation of the system was divided into four steps:
- preprocessing,
- anomaly detection,
- diagnosis and
- prognosis.
These steps are based on the current state of research on predictive maintenance and offer a rough roadmap of which steps should be implemented.
Devices are grouped in pre-processing so that an AI model is only responsible for devices with the same hardware and similar usage. In addition, relevant sensor data is selected depending on the application.
Anomaly detection uses a deep learning architecture to detect deviations from the device's normal state. In this way, it can be decided whether further actions are necessary for the identified device. In the diagnosis phase, the state of the device is decided, among other things through the use of expert knowledge. In this way, conditions shortly before the device fails can be reliably identified as such.
Finally, a prognosis of the device status is made. A suitable model is used to predict a trend that the device status may follow.
Main topics
There are two main research areas in this concept: Transfer Learning and Explainable AI.
A high data quality and quantity is a challenge for AI models in every real use case. Transfer learning attempts to balance an existing imbalance in the available information and to transfer knowledge from an already trained AI model to others.
In order for a predictive maintenance system to be used successfully in practice, future users must have a certain level of trust in the system. This trust is largely built up by the fact that the decisions of an AI model are comprehensible and reproducible. Explainable AI deals with this problem and creates trust in the future predictive maintenance system even for non-experts.
Conclusion
The PAS4PCM project is about researching predictive maintenance in relation to real use cases in Rational canteen kitchen appliances. Thanks to Rational's preliminary work, it is possible to build on a solid database and thus validate scientific concepts in practice. The concept offers a lot of space for interesting questions that are not only relevant for this project.
Contact
Internal Partner
Phone: | +49 821 5586-3445 |
Fax: | +49 821 5586-3499 |
External Partner
Rational Technical Services GmbH | |
Sven Walleser | |
Landsberg am Lech |
Funding
VDI/VDE-IT |
Bayerisches Verbundforschungsprogramm (BayVFP) des Freistaates Bayern | |
Förderlinie „Digitalisierung“ |